MMIS-2024@ACM MM 2024: Multi-rater Medical Image Segmentation for Radiotherapy Planning in Nasopharyngeal Carcinoma and Glioblastoma
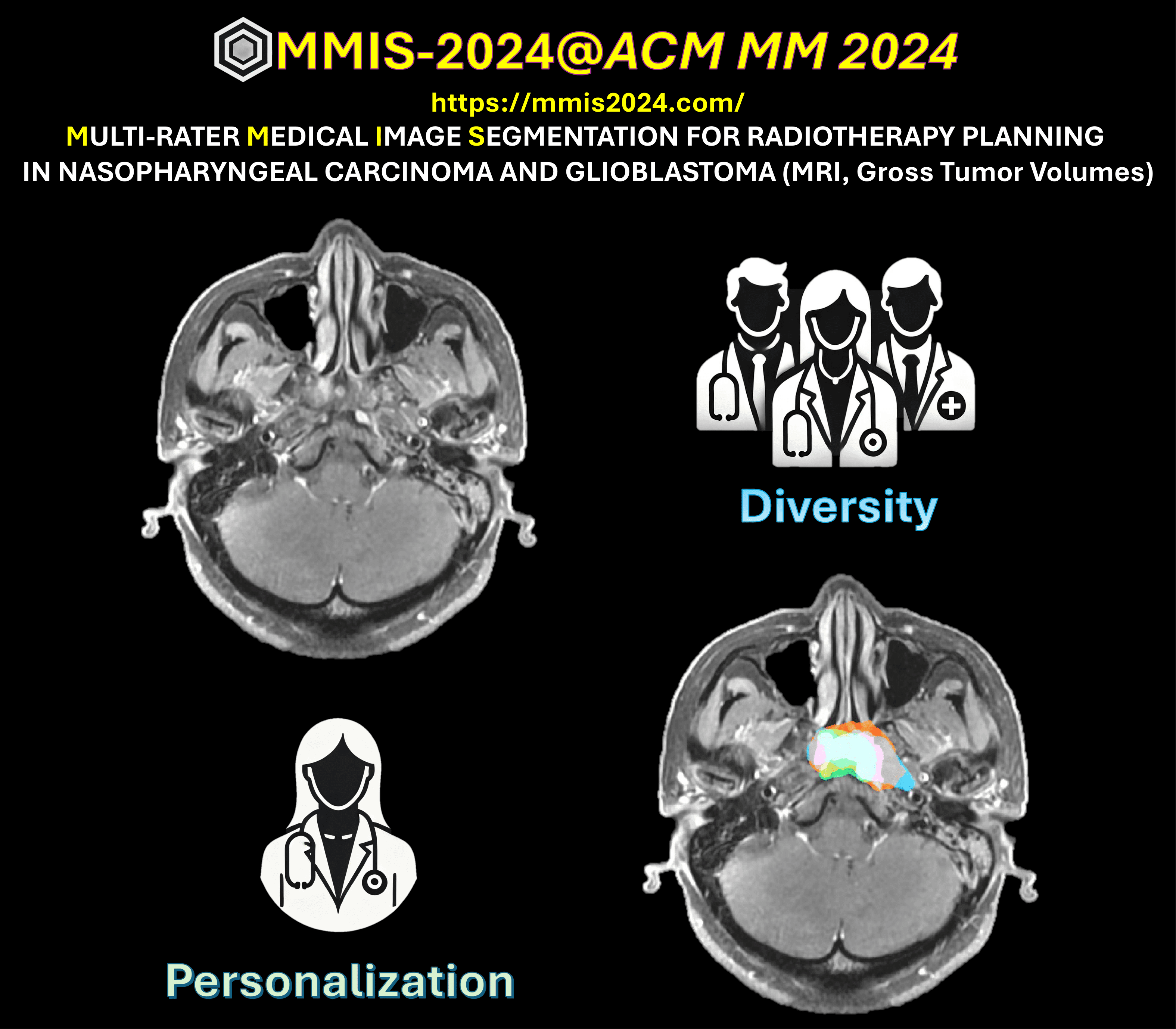
MMIS-2024 Challenge Program@ACM MM 24
Time slots | 10.28-Monday-MMIS 2024-Agenda | ||||
---|---|---|---|---|---|
9.00-9.10 | Opening (Yicheng Wu) | ||||
9.10-9.50 | Prof. Karen Caeyenberghs | Deakin University | On-site | Multi-center MRI studies in traumatic brain injury and how to deal with variability | Chair: Yicheng Wu |
9.50-10.30 | Dr. Chenyu Tim Wang | The University of Sydney | On-site | Developing medical imaging Al models for large-scale deployment: Expecting the unexpected | Chair: Yutong Xie |
10.30-11.00 | Morning Tea | ||||
11.00-11.40 | Dr. Dakai Jin | Alibaba DAMO Academy | Online | Continual Multi-Organ Segmentation Over Partially Labeled CT Datasets | Chair: Yicheng Wu |
11.40-11.50 | Challenge Details Introduction (Yutong Xie) | Chair: Yutong Xie | |||
11.50-12.00 | Jianghao Wu | UESTC-HiLab | Online | 1st Place | |
12.00-12.10 | Han Zhang | LKRobotAlLab | Online | 2nd Place | |
12.10-12.20 | Leilei Wang | USTC | Online | 3rd Place | |
12.20-12.30 | Award and Future Work (Yutong Xie) | ||||
Notes: A 30-min talk with 10-min QA; |
News
[2024.09.30] We start the second evaluation round of our challenge (due on October 24, 2024). During this phase, we will release the performance results for each instance to all participants. We have observed significant variation in performance across different instances and hope that this feedback will assist participants in refining their models.
[2024.08.15] We decide to host a half-day hybrid workshop during the ACM MM main conference. A detailed agenda will be shown soon.
[2024.08.15] There is a mistake in the old docker preparation. To simplify the evaluation process, we decide to release the testing data by emails. All participants are required to share their code and predictions with us. Specific requirements can be found in the Section-Evaluation.
[2024.07.23] We open the evaluation of task-2. See the Section-Evaluation for more details.
[2024.07.18] We open the evaluation of task-1. See the Section-Evaluation for more details.
[2024.07.01] We release the training set of task-2.
[2024.06.18] We release the training set of task-1.
[2024.06.17] We open the challenge website. Please check the timeline.
Overview
Image segmentation in the medical domain presents inherent subjectivity due to data-level ambiguities and diverse expert preferences. These factors lead to varying interpretations of the same target, limiting the deployment of AI models in clinical settings. This challenge invites you to address the multi-rater problem by focusing on the segmentation of diverse and personalized Gross Tumor Volumes (GTV) from MRI data, which is critical for radiotherapy planning in Nasopharyngeal Carcinoma and Glioblastoma.
Diverse segmentation offers a comprehensive view of possible interpretations, capturing a full range of expert opinions and enhancing the robustness of AI models in clinical decision-making. This approach ensures that critical variations in interpretation are not overlooked, which is particularly valuable in complex cases. Personalized segmentation, on the other hand, tailors the results to align with the specific preferences and methodologies of individual clinicians, resulting in more relevant treatment plans and reducing clinician workload. In clinical applications, these methods support improved radiotherapy planning by accommodating both the variability in expert assessments and the need for individualized treatment strategies.
Tasks
Task 1: Multi-Rater Medical Image Segmentation for Nasopharyngeal Carcinoma
(1) Each sample in this task is annotated by four different experts.(2) Participants are encouraged to utilize all annotations for model training.
Task 2: Federated Multi-Rater Medical Image Segmentation for Glioblastoma
(1) Each sample is annotated by a single expert.(2) Participants are encouraged to explore models by using only one expert annotation per sample.
Objectives
This challenge aims to enhance radiotherapy planning for Nasopharyngeal Carcinoma and Glioblastoma by developing AI models that can handle the complexity and variability of expert interpretations, ultimately leading to more personalized and effective treatment strategies.Dataset
To access the datasets of task 1, please do the registration first.
Task 2: We collated the glioblastoma imaging datasets from three publicly available repositories (i.e., LUMIERE, RHUH, UPENN-GBM) with postoperative and recurrence MRI scans. Each MRI study included four sequences: T1-weighted, T2-weighted, FLAIR, T1-weighted with gadolinium contrast. Four annotators (two radiation oncologists and two radiology residents) segmented tumours on the T1 contrast-enhanced sequence.
Training set: 120 scans were evenly split between the annotators i.e. 30 scans each.
Evaluation set: A further 20 scans were labelled by each annotator.
To access the datasets of task 2, please do the registration first.
Agenda
Event | Timeline | ||||
---|---|---|---|---|---|
Release of task-1 training/validation data | 06/18/2024 | ||||
Release of task-2 training/validation data | 07/01/2024 | ||||
First round of submission for task-1 evaluation | 07/18/2024-09/25/2024 | ||||
First round of submission for task-2 evaluation | 08/01/2024-09/25/2024 | ||||
Second round of evaluation | 09/25/2024-10/24/2024 | ||||
Announcement of final leaderboards | 10/26/2024 | ||||
Onsite Challenge | 10/28/2024 | ||||
Prizes
1. Cash awards for each task ($1800 USD in total):First Prize: $500
Second Prize: $300
Third Prize: $100
2. Certificates for the top-rank teams.
3. Besides, there will be a challenging paper, which will be submitted to a top-tier journal (e.g., MedIA/TMI). Top rankers will be invited as co-authors of the challenging paper.
Evaluation
For evaluation, we follow Wu_2024_CVPR, Luo_2023_deep to do the evaluation for multi-rater medical image segmentation. In general, we evaluate the segmentation results from two aspects:1. Diverse performance: we use the GED score, Dicesoft score, and two set-level metrics DiceMatch and DiceMax to compare the similarity between the results set and label set.
2. Personalized performance: we compute the Dice score for each individual expert and then use their mean value as the final metric.
For each task, we will use a combination score of these metrics for the final ranking. All participants are required to submit their code, model weights, and predictions with us.
If you are ready to submit your results, please email them to mmis2024acm@gmail.com with the subject “[Team_Name]-[Task_1/2]-[Submission Time]”. After manual evaluation, your ranking will be displayed on the leaderboard. Note that each team has only three opportunities to submit their model. Good luck!
Task-1
For Task 1, the input directory is /Images/Testing/. Each sample is an h5 file containing three modalities: “t1”, “t1c”, and “t2”. Please note that no labels are provided in the test set. We require all the teams to provide 30 diversified segmentation results in /Output/Diversified/ and 4 corresponding expert predictions in /Output/Personalized/ for each sample.
MMIS2024_Task1_Example
• Images
• Testing
• Sample_0.h5, …, Sample_49.h5
• Model
• [your own model and weights]
• Output
• Diversified
• Sample_0
• Seg1.nii.gz, …, Seg30.nii.gz
• …
• Sample_49
• Seg1.nii.gz, …, Seg30.nii.gz
• Personalized
• Sample_0
• Pred_1.nii.gz, …, Pred_4.nii.gz
• …
• Sample_49
• Pred_1.nii.gz, …, Pred_4.nii.gz
Task-2
For Task 2, the input directory is /MMIS2024TASK2_test/. Each MRI study in test set included four sequences: T1-weighted, T2-weighted, FLAIR, T1-weighted with gadolinium contrast. Please note that no labels are provided in the test set. We encourage teams to provide 30 diversified segmentation results in /Output/Diversified/ and 4 corresponding expert predictions in /Output/Personalized/ for each sample.
MMIS2024_Task2_Example
• MMIS2024TASK2 test
• Sample_0
• T1contrast.nii.gz, T1.nii.gz, T2.nii.gz, FLAIR.nii.gz
• …
• Sample_19
• T1contrast.nii.gz, T1.nii.gz, T2.nii.gz, FLAIR.nii.gz
• Model
• [your own model and weights]
• Output
• Diversified
• Sample_0
• Seg1.nii.gz, …, Seg30.nii.gz
• …
• Sample_19
• Seg1.nii.gz, …, Seg30.nii.gz
• Personalized
• Sample_0
• Pred_1.nii.gz, …, Pred_4.nii.gz
• …
• Sample_19
• Pred_1.nii.gz, …, Pred_4.nii.gz
•Yicheng Wu, Monash University, Australia
•Yutong Xie, Australian Institute for Machine Learning (AIML), University of Adelaide, Australia
•Xiangde Luo, Sichuan Cancer Hospital & Institute, Sichuan Cancer Center, China.
•Wenjun Liao, Sichuan Cancer Hospital & Institute, Sichuan Cancer Center, China.
•Minh-Son To, Flinders Health and Medical Research Institute, Flinders University, Australia
•Peter Gorayski, Australian Bragg Centre for Proton Therapy, South Australia Health and Medical Research Institute, Australia
•Hien Le, Australian Bragg Centre for Proton Therapy, South Australia Health and Medical Research Institute, Australia
•Adon Toru Asahina, Flinders Health and Medical Research Institute, Flinders University, Australia
•Jianfei Cai, Monash University, Australia
•Qi Wu, Australian Institute for Machine Learning (AIML), University of Adelaide, Australia
•Weidong Cai, University of Sydney, Australia
•Zhaolin Chen, Monash University, Australia
•Yong Xia, Northwestern Polytechnical University, China
•Zihao Tang, University of Sydney, Australia






Citations
2. Wu, Y., Luo, X., Xu, Z., Guo, X., Ju, L., Ge, Z., Liao, W., Cai, J.: Diversified and personalized multi-rater medical image segmentation. In: Proceedings of the IEEE/CVF Conference on Computer Vision and Pattern Recognition (CVPR). pp. 11470–11479 (June 2024)
3. Bakas, S., Sako, C., Akbari, H., Bilello, M., Sotiras, A., Shukla, G., Rudie, J. D., Flores Santamaria, N., Fathi Kazerooni, A., Pati, S., Rathore, S., Mamourian, E., Ha, S. M., Parker, W., Doshi, J., Baid, U., Bergman, M., Binder, Z. A., Verma, R., … Davatzikos, C. (2021). Multi-parametric magnetic resonance imaging (mpMRI) scans for de novo Glioblastoma (GBM) patients from the University of Pennsylvania Health System (UPENN-GBM) (Version 2) [Dataset]. The Cancer Imaging Archive. https://doi.org/10.7937/TCIA.709X-DN49
4. Cepeda, S., García-García, S., Arrese, I., Herrero, F., Escudero, T., Zamora, T., & Sarabia, R. (2023). The Río Hortega University Hospital Glioblastoma dataset: A comprehensive collection of preoperative, early postoperative and recurrence MRI scans (RHUH-GBM). In Data in Brief (Vol. 50, p. 109617). Elsevier BV. https://doi.org/10.1016/j.dib.2023.109617
5. Cepeda, S., García-García, S., Arrese, I., Herrero, F., Escudero, T., Zamora, T., & Sarabia, R. (2023) The Río Hortega University Hospital Glioblastoma dataset: a comprehensive collection of preoperative, early postoperative and recurrence MRI scans (RHUH-GBM) [Dataset]. The Cancer Imaging Archive. https://doi.org/10.7937/4545-c905
6. Suter, Y., Knecht, U., Valenzuela, W. et al. The LUMIERE dataset: Longitudinal Glioblastoma MRI with expert RANO evaluation. Sci Data 9, 768 (2022). https://doi.org/10.1038/s41597-022-01881-7
Terms and Conditions
2. No additional data is allowed. We will require all participants to provide extensive documentation on their development processes, including data and methods used. Top-ranked participants must submit their training codes and checkpoints for verification. Publicly available pre-trained models are allowed, ensuring a level playing field and transparency in the competition's evaluation process.
3. The top-ranking teams will be notified two weeks before the challenge day to prepare their presentations. Final results and awards will be announced on the challenge day.
4. Independent Publications: Participating teams are allowed to publish their results separately. However, this should be done in a manner that respects the collective efforts of the challenge.
5. Embargo Period: An embargo period allows the challenge organizers to publish a comprehensive challenge paper first. During this period, individual teams are encouraged to refrain from publishing their complete findings independently.